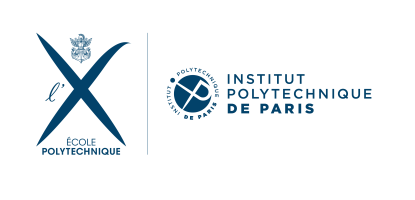
Log in to École polytechnique learning platform
Is this your first time here?
Première visite sur ce site ? First time on our website ?
If you are currently enrolled at École Polytechnique, please enter your LDAP login FIRSTNAME.LASTNAME (SynapseS, computer rooms, email, etc.) and not an email.
Si vous êtes déjà inscrit à l’École Polytechnique, merci d’entrer votre login LDAP PRENOM.NOM (SynapseS, salles info, messagerie, etc.) et non un email.
If you are a prospective student, please click on “connexion anonyme” or “log in as a guest” below on the left section.
Si vous êtes candidat à un programme de l’École, merci de cliquer sur “connexion anonyme” en bas à gauche.